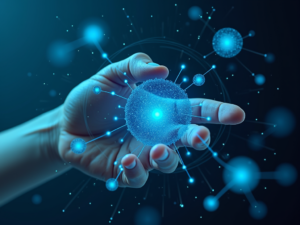
Artificial intelligence (AI) is poised to revolutionize diabetes care by introducing data-driven precision, personalized treatment strategies, and scalable solutions to address the growing global burden of the disease. By integrating machine learning algorithms, continuous glucose monitoring (CGM), and telemedicine platforms, AI enhances glycemic control, reduces complications, and empowers patients through real-time insights. This report synthesizes advancements in AI-driven diagnostics, predictive analytics, and automated insulin delivery systems, while addressing ethical, regulatory, and implementation challenges shaping the future of diabetes management.
AI-Driven Personalized Treatment Planning
Tailoring Interventions Through Multidimensional Data Analysis
AI systems analyze clinical records, genetic profiles, and lifestyle data to generate personalized dietary, exercise, and pharmacological recommendations. For instance, machine learning models combine electronic health records (EHRs) and real-time glucose data to optimize meal plans and insulin dosages24. A study in China demonstrated that AI-integrated platforms reduced HbA1c levels by 1.2% in adults with type 2 diabetes (T2D) through customized dietary guidance linked to CGM feedback2. Similarly, the “FoodLens” AI tool identifies food types from images, enabling patients to adjust carbohydrate intake dynamically based on glucose trends23.
Precision in Pharmacological Management
AI enhances insulin therapy by automating dose adjustments via smart pens and closed-loop systems. Hybrid closed-loop algorithms, such as those in the MiniMed™ 780G system, adjust basal rates and administer correction boluses every five minutes, mitigating postprandial hyperglycemia12. Trials show these systems improve time-in-range (70–180 mg/dL) by 15% while reducing hypoglycemic events712. For non-insulin therapies, AI predicts drug efficacy using biomarkers like fasting glucose and BMI, enabling clinicians to prioritize GLP-1 agonists or SGLT2 inhibitors based on individual risk profiles914.
Complication Prevention and Targeted Therapies
Deep learning algorithms detect early signs of diabetic retinopathy from fundus images with 98% accuracy, enabling timely interventions28. Similarly, AI models analyzing urinary albumin-to-creatinine ratios and blood pressure trends predict nephropathy progression, allowing preemptive use of ACE inhibitors39. These tools shift care from reactive to preventive paradigms, reducing long-term morbidity16.
Real-Time Monitoring and Early Warning Systems
Integration with Wearable Technologies
AI-powered CGM devices, such as the Eversense system, provide non-invasive glucose monitoring through subcutaneous sensors and optical spectroscopy, offering 90-day continuous data with 11.4% MARD accuracy212. Coupled with smartwatches tracking heart rate variability and activity levels, these systems predict hypoglycemia 60 minutes in advance using recurrent neural networks (RNNs)27. The Accu-Chek SmartGuide CGM solution exemplifies this, alerting users to impending hyperglycemia based on glucose velocity and meal absorption patterns515.
Predictive Analytics for Acute Events
Machine learning models like random forests and long short-term memory (LSTM) networks analyze historical glucose patterns, physical activity, and stress markers to forecast severe hypoglycemia (≤54 mg/dL) with 92% sensitivity27. In the Utah Remote Monitoring Project, AI-driven alerts reduced emergency hospitalizations by 34% among high-risk T2D patients14. Non-invasive sensors using saliva and tear fluid biomarkers further expand monitoring accessibility, particularly in resource-limited settings214.
Dynamic Risk Stratification
AI classifies patients into risk tiers using variables such as glycemic variability, lipid profiles, and socioeconomic factors. A multimodal model combining retinal scans and EHRs predicted cardiovascular events in T2D patients with an AUC of 0.89, outperforming traditional Framingham scores914. These stratification tools enable targeted resource allocation, prioritizing high-risk individuals for intensive interventions39.
Telemedicine and Decentralized Health Management
Virtual Care Platforms and Remote Consultations
AI-driven telemedicine platforms like DeepDR-LLM provide real-time decision support to primary care providers, improving adherence to diabetes guidelines by 40% in rural China28. Video consultations integrated with CGM data allow endocrinologists to adjust insulin regimens remotely, reducing clinic visits by 50% while maintaining glycemic targets214. In the U.S., the Guardian Connect System alerts clinicians to abnormal glucose trends, enabling proactive dose adjustments via secure messaging1215.
Patient Empowerment Through Digital Therapeutics
Mobile apps employing reinforcement learning deliver personalized behavioral nudges, such as post-meal walking reminders based on glucose spikes. A trial using the Dario app demonstrated a 0.8% reduction in HbA1c among T2D patients through gamified lifestyle tracking15. Social media communities like #t1d on Twitter foster peer support, while anonymized patient data from these platforms train AI models to identify barriers to medication adherence27.
Scalability in Low-Resource Settings
In India, AI-powered chatbots provide dietary advice in regional languages, addressing the shortage of diabetes educators. A pilot in Maharashtra improved glycemic control in 62% of users by combining SMS-based education with AI-analyzed glucose logs14. Similarly, Kenya’s M-Tiba platform uses blockchain-secured AI to coordinate care between patients, pharmacists, and providers, reducing insulin stockouts by 75%614.
Clinical and Operational Effectiveness of AI Solutions
Enhanced Glycemic Control and Complication Reduction
Meta-analyses of 40 studies show AI interventions reduce HbA1c by 0.5–1.5% compared to standard care, with the largest improvements seen in closed-loop insulin systems37. The IDx-DR AI system slashed diabetic retinopathy screening costs by 60% while increasing detection rates by 22% in U.S. primary care clinics89. Automated retinal imaging analysis alone could prevent 120,000 blindness cases annually in low-income countries68.
Cost Savings and Workflow Optimization
AI reduces clinician workload by automating routine tasks like glucose data interpretation and prior authorization. At Mayo Clinic, natural language processing (NLP) tools extract diabetes-related variables from unstructured EHR notes in 12 seconds, down from 15 minutes manually39. Predictive discharge algorithms shortened hospital stays for diabetic ketoacidosis (DKA) by 1.2 days, saving $3,200 per admission1415.
Improving Medication Adherence and Self-Efficacy
AI-enhanced apps tracking pill ingestion via smartphone cameras boosted adherence to oral hypoglycemics by 35% in a 2024 U.S. trial14. Personalized text reminders informed by machine learning—considering factors like health literacy and cultural preferences—increased metformin adherence by 28% among Latino patients1415. These tools bridge gaps in traditional care models, particularly for marginalized populations69.
Ethical, Legal, and Regulatory Challenges
Data Privacy and Security Risks
Centralized AI platforms aggregating sensitive health data face cyberattack vulnerabilities; a 2024 breach at a European telehealth firm exposed 230,000 diabetes patients’ CGM records613. Differential privacy techniques, which add statistical noise to datasets, are being adopted to anonymize information while preserving analytical utility913. The EU’s AI Act mandates encryption of biometric data, requiring developers to conduct third-party audits for compliance1314.
Algorithmic Bias and Health Inequities
Studies reveal racial disparities in AI performance; a deep learning model for retinopathy screening had 79% sensitivity in Black patients versus 94% in White cohorts due to underrepresentation in training data914. Mitigation strategies include federated learning across diverse populations and fairness-aware algorithms that adjust for socioeconomic confounders39. India’s 2023 Digital Health Law mandates demographic parity in AI training datasets to prevent caste-based biases14.
Regulatory Frameworks and Liability Concerns
The FDA’s 2025 draft guidance on AI lifecycle management requires continuous post-market surveillance, necessitating real-world performance tracking through PCCPs (Predetermined Change Control Plans)1113. Health Canada’s 2024 regulations hold manufacturers liable for algorithmic drift causing patient harm, exemplified by a 2023 recall of an insulin dosing app after unapproved updates led to dosing errors1114. Global harmonization remains elusive, complicating multinational deployments of AI tools1314.
Future Prospects and Innovations
Predictive Prevention and Population Health
AI-driven risk scores using polygenic risk markers and environmental data could identify prediabetic individuals five years before onset, enabling lifestyle interventions to reduce progression by 58%915. Sanofi’s partnership with Owkin employs federated learning to analyze 450,000 EHRs, identifying novel T2D subtypes for targeted prevention15. Municipalities in Finland use AI to optimize green spaces and walkability, projected to lower diabetes incidence by 9% by 203015.
Next-Generation Closed-Loop Systems
Fully autonomous “artificial pancreases” integrating CGM, insulin pumps, and glucagon delivery are undergoing trials. The Beta Bionics iLet system, using reinforcement learning, achieved 80% time-in-range without meal announcements in a 2025 T1D study1012. For T2D, Medtronic’s investigational system combines closed-loop insulin with GLP-1 microdosing, showing 1.8% HbA1c reductions in obese patients1215.
AI-Powered Drug Discovery and Precision Medicine
Generative AI models like AlphaFold 3 are designing novel dual GIP/GLP-1 agonists with enhanced beta-cell regenerative properties. Insilico Medicine’s AI-discovered molecule, ISM049-055, entered Phase II trials in 2025, targeting insulin resistance pathways specific to South Asian genomes1415. Pharmacogenomic algorithms now guide metformin prescribing based on SLC22A1 variants, reducing gastrointestinal side effects by 44%914.
Global Integration and Health System Resilience
The WHO’s 2025 AI Governance Framework advocates for equitable access, prioritizing low-cost solutions like AI-optimized insulin titration charts for community health workers1315. In sub-Saharan Africa, drone-delivered AI diagnostics reduced diabetic foot ulcer amputations by 30% through early detection614. However, achieving universal adoption requires addressing infrastructural gaps—only 35% of LMICs have the broadband capacity for real-time AI analytics1415.
Conclusion
AI is redefining diabetes care through hyper-personalized treatments, proactive complication prevention, and democratized access to expertise. While challenges like algorithmic bias and regulatory fragmentation persist, collaborative efforts among clinicians, policymakers, and technologists are driving solutions that balance innovation with equity. As closed-loop systems and AI-driven drug discovery mature, the vision of zero preventable diabetes complications becomes increasingly attainable. Future success hinges on global data-sharing frameworks, culturally adapted tools, and sustained investment in AI literacy among providers and patients alike.
Citations:
- https://pubmed.ncbi.nlm.nih.gov/32325045/
- https://pmc.ncbi.nlm.nih.gov/articles/PMC11718508/
- https://pmc.ncbi.nlm.nih.gov/articles/PMC10789841/
- https://www.taylorfrancis.com/chapters/edit/10.1201/9781003522096-6/future-diabetic-management-using-artificial-intelligence-ajay-sharma-shubhra-dixit-surbhi-gupta
- https://thejournalofmhealth.com/using-ai-to-transform-diabetes-management/
- https://esmed.org/MRA/mra/article/view/4287
- https://diatribe.org/diabetes-technology/how-artificial-intelligence-changing-diabetes-care
- https://diabetesvoice.org/en/caring-for-diabetes/is-ai-the-future-of-diabetes-diagnosis-and-management/
- https://www.nature.com/articles/s41746-024-01034-7
- https://health.ucdavis.edu/news/headlines/with-ai-a-new-metabolic-watchdog-takes-diabetes-care-from-burden-to-balance/2025/02
- https://www.medtechdive.com/news/fda-health-canada-future-ai-regulations/729953/
- https://www.nature.com/articles/d42473-021-00265-6
- https://www.nature.com/articles/s41599-024-02894-w
- https://journals.sagepub.com/doi/10.1177/23333928241275292?icid=int.sj-full-text.similar-articles.3
- https://www.sanofi.com/en/magazine/your-health/the-digital-future-of-diabetes-care
- https://www.thelancet.com/journals/landia/article/PIIS2213-8587(24)00154-2/fulltext
- https://www.york.ac.uk/news-and-events/news/2024/research/ai-diabetes-care-bradford-patients/
- https://www.schulich.uwo.ca/research/about_us/monthly_enewsletter/2020/march/research_diabetes_and_ai.html
- https://www.linkedin.com/pulse/how-ai-transforming-diabetes-care-diabetotech-bw8oe
- https://www.bcg.com/publications/2025/digital-ai-solutions-reshape-health-care-2025
- https://www.euractiv.com/section/health-consumers/news/a-massive-diabetes-challenge-and-pharma-tech-moonshots-europe-starts-2025-in-launch-mode/
- https://www.thelancet.com/journals/landia/article/PIIS2213-8587(24)00154-2/abstract
- https://www.marctech2.com/news/wearable-health-tech-in-2025-potential-innovations-shaping-the-future-of-diabetes-management
- https://www.idtechex.com/en/research-report/diabetes-management-technologies-2025-2035-markets-players-and-forecasts/1059
- https://diabetesjournals.org/care/article/48/2/182/157738/Large-Language-Models-in-Diabetes-Management-The
- https://www.roche.com/stories/advancing-diabetes-care
- https://www.linkedin.com/pulse/11-companies-shaping-future-diabetes-more-news-health-mesk%C3%B3-md-phd-qldde
- https://www.uab.edu/reporter/patient-care/advances/item/10135-diabetes-technology-the-future-is-today
- https://www.thebusinessresearchcompany.com/report/artificial-intelligence-in-diabetes-management-global-market-report
- https://ictandhealth.com/news/advances-in-ai-for-life-sciences-to-be-expected-in-2025
- https://jamanetwork.com/journals/jama/fullarticle/2830340
- https://srinstitute.utoronto.ca/news/transforming-diabetes-care
- https://www.ajmc.com/view/2025-outlook-tackling-ai-cybersecurity-and-regulatory-challenges
- https://www.thelancet.com/journals/landig/article/PIIS2589-7500(24)00143-2/fulltext
- https://jamanetwork.com/journals/jama-health-forum/fullarticle/2823255
- https://www.ama-assn.org/practice-management/digital/advancing-health-care-ai-through-ethics-evidence-and-equity
- https://iris.who.int/bitstream/handle/10665/341996/9789240029200-eng.pdf
- https://formative.jmir.org/2025/1/e57874
- https://www.niddk.nih.gov/news/meetings-workshops/2024/artificial-intelligence-in-precision-medicine-of-diabetes-2024
- https://www.news-medical.net/health/Ethical-Considerations-in-AI-Driven-Healthcare.aspx